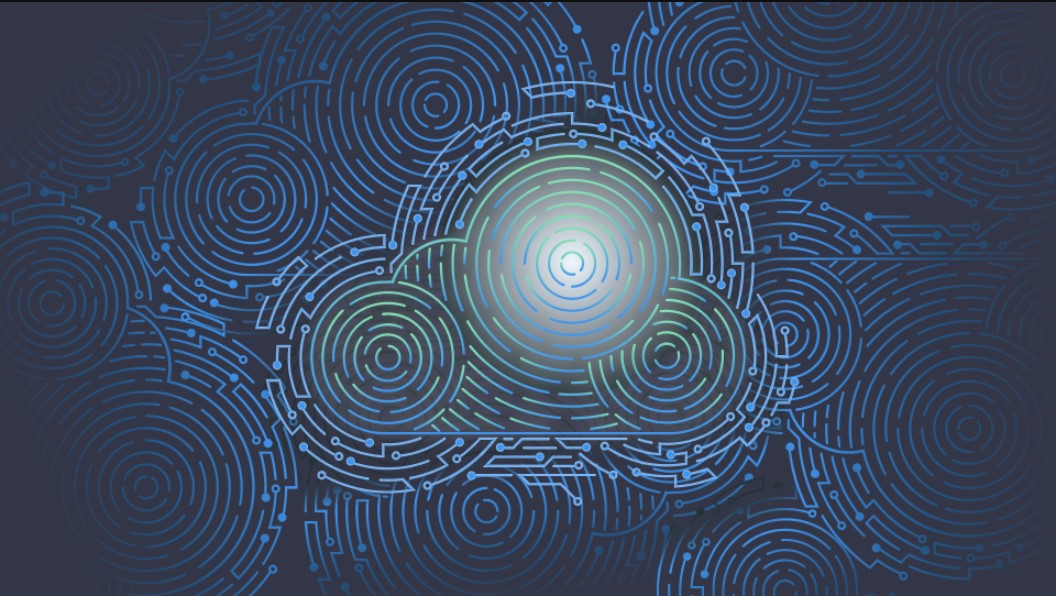
In today’s data-driven economy, artificial intelligence (AI) is no longer a futuristic concept — it’s an operational reality. From self-optimizing supply chains to personalized customer experiences, AI-powered systems are now at the core of digital transformation initiatives. But while the spotlight often falls on training sophisticated AI models, the real value is delivered during inference — the moment a model applies its learned intelligence to generate insights or predictions.
This is where AI Inference as a Service (AI IaaS) steps in. Offering flexible, scalable, and optimized environments for model deployment, AI IaaS is empowering businesses to transform AI from an experimental asset into a production-grade solution — without the complexity of managing hardware or fine-tuning infrastructure.
What is AI Inference as a Service?
AI Inference as a Service refers to cloud-based platforms that host pre-trained machine learning (ML) or deep learning models and deliver real-time predictions through APIs or other standardized interfaces. Once a model has been trained, inference is the process of using that model to process new data and produce outcomes — whether it’s recommending products, detecting anomalies, or interpreting images.
Historically, deploying inference workloads at scale required specialized hardware, complex configuration, and significant operational overhead. AI IaaS abstracts this complexity by offering a ready-made, pay-as-you-go environment where enterprises can deploy models and immediately access production-ready inference capabilities.
Why AI Inference as a Service is Gaining Momentum
Several trends are driving the rapid adoption of AI IaaS:
1. Speed to Market
In competitive industries, the ability to rapidly operationalize AI models can make or break a project. AI IaaS platforms eliminate the need for infrastructure provisioning, offering pre-optimized pipelines that accelerate deployment from months to days.
2. Cost Optimization
Inference workloads can fluctuate dramatically depending on business needs. Cloud-based inference services offer elastic scaling and usage-based pricing, ensuring businesses only pay for the compute resources they consume, avoiding costly idle infrastructure.
3. Performance and Scalability
AI IaaS providers often leverage high-performance computing resources like GPUs, TPUs, and AI-optimized chips. This allows businesses to handle everything from low-latency, real-time inference to large-scale batch predictions without bottlenecks.
4. Simplified Maintenance
Model updates, security patches, and performance tuning can require extensive DevOps effort in on-prem environments. AI IaaS providers handle these challenges behind the scenes, freeing teams to focus on refining models and business strategy.
Practical Applications Across Industries
The versatility of AI IaaS is reshaping industries in measurable ways:
-
Healthcare: AI-driven diagnostics tools use inference services to analyze X-rays and MRIs in real-time, aiding clinicians in faster decision-making.
-
Finance: Fraud detection systems continuously evaluate transaction patterns through AI IaaS platforms, flagging suspicious activity almost instantly.
-
E-commerce: Recommendation engines use inference services to personalize customer experiences based on user behavior and historical data.
-
Manufacturing: Predictive maintenance models leverage inference platforms to identify equipment anomalies before failure occurs.
Considerations Before Adoption
While AI IaaS offers remarkable advantages, businesses should evaluate:
-
Data Security: Ensure providers meet necessary data compliance standards (HIPAA, GDPR, ISO/IEC 27001) especially when handling sensitive data.
-
Latency Requirements: For real-time applications, verify the geographic distribution and edge computing capabilities of the service provider.
-
Vendor Flexibility: Open-source compatibility and support for multiple frameworks like TensorFlow, PyTorch, and ONNX can protect against vendor lock-in.
A Future Powered by AI Inference
As AI continues to evolve from research labs to enterprise infrastructure, inference workloads are expected to surpass training workloads in both volume and business impact. With emerging technologies like edge computing and AI cloud model compression, inference will become even faster, cheaper, and more accessible.
The rise of AI Inference as a Service is not just about operational convenience — it’s a signal that businesses must rethink how intelligence is delivered, consumed, and monetized.
Final Takeaway
In a world where speed, agility, and intelligence are the new currency, AI Inference as a Service offers a strategic advantage that organizations cannot afford to ignore. By offloading infrastructure concerns and focusing on delivering value from AI insights, businesses can shift from reactive decision-making to predictive innovation — and position themselves for lasting success in the age of AI.