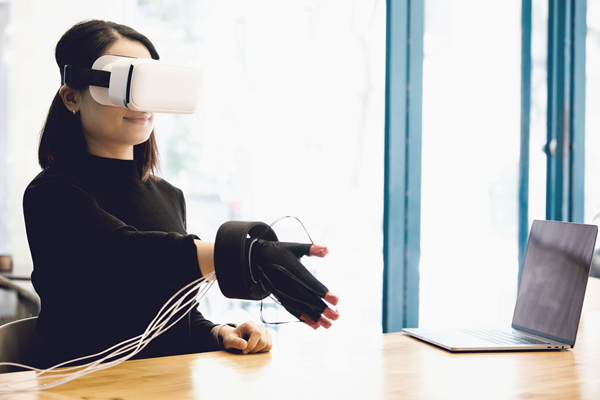
Artificial intelligence (AI) can have a transformative impact on healthcare, like improving patient outcomes and clinical trials. It is a real struggle to bring new drugs and clinical therapies from the lab to the market, as it takes 8 to 12 years and is expensive. Inefficiencies in the clinical trial and development are the primary obstacles. Despite the implementation of various strategies to overcome the obstacle, the success rate of clinical drug development is relatively low. Moreover, trials often exclude marginalized populations, highlighting the inequality in healthcare delivery.
Generative AI technology offers the opportunity to help clinical development teams design better and more efficient trials, such as decentralized clinical trials (DCTs) and improve the existing designs by integrating new data streams. So, with smart investments and an enabling and supporting environment, Gen AI can enhance the development of trial design, improve the trial feasibility and site selection. Gen AI also provides new avenues for clinical research, based on real-time evidence, adaptive designs and continuous learning. According to many clinical development experts, five processes have the biggest potential for transformation through Gen AI.
Clinical trial design
The clinical trial design currently is a complex process that involves designing detailed protocols. These protocols can significantly impact the success of a trial, but they often lead to inefficiencies due to slow manual workflows and reliance on subjectivity.
Generative AI possesses the transformative potential to mine unstructured data from previous protocols, real-world data (RWD), regulatory guidance, and patient feedback to optimize the trial design. An AI consulting company can assist the development team in the following ways:
- Streamline trial design and protocol drafting by automating traditional manual processes.
- Simulate scenarios to identify the most effective designs.
- Create digital and surrogate endpoints, synthetic control arms, and in silico trials.
- Unlock greater predictive power using unstructured data.
Through these tasks, Gen AI can reduce errors, accelerate trial imitation, reduce costs and increase the probability of success.
Trial feasibility and site selection
Currently, trial feasibility assessments and site selection are often ad hoc and reliant on historical data. Due to the inefficiencies in these processes, there has been under-enrollment in 85% of trials, costing the industry millions per day in missed market opportunities. Sites are chosen based on past experiences, such as site capacity and enrollment rates. The flaw in this process is that it often overlooks local patient demographics, disease prevalence and trial-specific site capabilities.
Other barriers faced in trial feasibility include fragmentation of health data and lack of standardized data and data-sharing practices. Generative AI can help in developing robust data integration standards and enhanced regulatory frameworks. It can analyze operational and unstructured data from past trials and real-time evidence. So, Generative AI facilitate real-time modeling that predicts potential challenges at different sites before trials even begin.
- Predict patient recruitment potential at various sites.
- Suggest optimal sites based on local demographics, disease prevalence and site capabilities.
- Simulate scenarios to address recruitment challenges proactively.
- Decentralize trials to make them more patient-centric.
These capabilities enable data-driven decisions, reduce delays and make the process more patient-centric.
Patient recruitment and retention
Traditional recruitment methods encounter certain challenges due to their slowness, inefficiency and inability to cater to trials with narrow inclusion criteria. Retention efforts often fail due to poor communication, logistical hurdles and the burden of participation.
Digital tools help reduce the challenges participants face in clinical trials, such as time commitments, disruptions in daily life, and financial costs. However, many people still dropout. We rarely use technology to identify potential dropouts or develop customized retention tactics. Utilizing an AI consulting company experienced in generative AI technology can further enrich these efforts by integrating advanced algorithms into existing frameworks. So, generative AI has the potential to revolutionize recruitment and retention.
- Automating recruitment workflows and consent form completion
- Analyzing data from electronic health records (EHRs), insurance claims and patient advocacy groups to reach diverse populations
- Using unstructured data to feed predictive models assessing the likelihood of participant dropout
- Tailoring personalized engagement strategies to anticipate dropout risks and recommend next-base actions
These improvements enhance the participant experiences, boost retention rates and minimize delays in trials.
Data analysis
The current challenge in data analysis is the integration and cleaning of data from multiple sources is time-intensive, error-prone and expensive. Complex data analysis slows down the process of real-time decision-making. Analysts work with different types of data like medical records, lab results, and patient reports, but these data sources don’t always fit together easily. Development teams face growing challenges related to data quality and standardization, as it requires substantial time and specialized expertise. Integrating machine learning models further enhances predictive analytics in clinical settings. Traditionally, data analysis has been labor-intensive, requiring numerous hours of sifting through vast datasets. Generative AI streamlines this task by automating the extraction and synthesis of relevant information.
Gen AI can act as a co-pilot in data analysis by:
- Automating data cleaning, integration and validation
- Generating code and statistical analysis scripts
- Reducing the time between data collection and informed-decision making
- Unlocking insights by integrating diverse data sources, like sensor data from wearables and patient-reported outcomes
This approach reduces the time between data collection and actionable decision-making, enabling faster, and more accurate analysis.
Regulatory submission and review
The regulatory submission is for the most part manual, leading to frequent errors, duplication of efforts and inefficiencies. Furthermore, the complexity of clinical trials has increased over the past decade, and the duration of trial submissions has also increased.
In the future, Gen AI has the potential to streamline regulatory submissions.
- Automating the compilation, organization and validation of submission of documents
- Ensuring compliance with varying regulatory requirements
- Using predictive algorithms to assess the likelihood of regulatory success
This lessens the workload for submission teams, eliminates the need for expensive requests for additional information, and expedites the approval process.
Generative AI has the potential to transform clinical trials from design to regulatory submission. They can automate the manual processes, enhance data-driven decisions and personalize the participant experiences. This can significantly reduce costs, accelerate timelines and improve outcomes. Stakeholders can collaborate to address the barriers by integrating Gen AI in clinical development, which could ensure faster access to life-saving therapies for patients worldwide.
Author Bio
The author is an experienced healthcare consultant specializing in the integration of advanced technologies like AI and machine learning in the healthcare industry. With a strong background in digital health transformation, he focuses on leveraging generative AI to enhance patient care, streamline healthcare processes, and address systemic challenges in the industry. He has authored and written for many healthcare journals, industry blogs and articles.