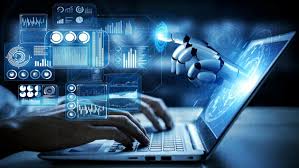
Artificial intelligence (AI) automation has the potential to transform companies-efficiency, data-driven insights, and cost reductions, to be specific. But when companies move from pilot projects to full-scale adoption, they run into roadblocks that challenge their capacities to transform promises into actuality. These are the most relevant issues with AI business automation at present and how to tackle them.
1. Data Quality: The Key to Successful AI
Data that is of poor quality-incomplete, inconsistent, and biased-results in defective predictions and inefficiencies in operations. A simple illustration is a bank that employs AI to evaluate credit risk: it may incorrectly categorize borrowers when historical data is unrepresentative or flawed.
Solutions:
Institute stringent data governance standards to maintain accuracy and completeness.
Apply synthetic data augmentation to complete gaps within datasets.
Regular audit of datasets for detecting and eliminating bias.
2. Integration with Legacy Systems
Many companies find it difficult to implement AI on legacy IT infrastructure. Legacy systems usually have no compatibility with new AI tools, which results in isolated workflows and bottlenecks. A retailer, for example, may discover that its stock management software is not compatible with AI-based demand forecasting models.
Solutions:
Focus on API-based AI solutions for smooth integration.
Gradually modernize infrastructure using cloud platforms for scalability.
Work with AI experts to close technology gaps.
3. Ethical and Compliance Risks
The “black box” character of AI is a source of ethical concern, especially over bias and explainability. Racial discrimination from biased hiring software or exclusionary loan granting algorithms could jeopardize reputations and create legal sanctions. Meta was charged a record-breaking $1.3 billion fine for GDPR infringements in 2022, highlighting the price of non-adherence.
Solutions:
Implement explainable AI (XAI) frameworks to make decision-making transparent.
Implement ethics committees for AI deployment.
Align AI practices with regulations such as GDPR and CCPA
4. Talent Shortages and Skill Gaps
Lack of expertise with AI is still a major hindrance. A survey in 2025 reveals that 65% of organizations point to talent gaps as a key roadblock that makes them choose between expensive externalization or labor-intensive internal training.
Solutions:
Upskill employees with workshops and certification programs.
Work with AI consultancies on temporary projects.
Develop partnerships with universities to create talent pipelines.
5. Resistance to Change from Employees
Employees may fear that AI would replace jobs or make workflows unnecessarily complicated. For instance, medical personnel may be opposed to AI-based diagnostics because they fear losing control.
Solutions:
Explain AI’s position as an assistant for choice-making, not a substitution.
Encourage employees’ involvement in implementing AI to establish trust.
Emphasize efficiency improvements, such as eliminating repetitive work
6. Scaling AI Beyond Pilots
Whereas 72% of companies implement AI for at least one function, enterprise-wide scale is elusive. A manufacturing company’s pilot for predictive maintenance can fail when scaled up because of the overload of information or lack of necessary infrastructure.
Solutions:
Start with department-specific pilots before scaling.
Invest in flexible cloud infrastructure to manage escalating data needs.
Continuously monitor performance and adjust models.
7. Setting Clear Objectives
Most AI projects fail due to imprecise objectives such as “improve customer experience”. A telecommunications company, for example, may squander resources implementing AI without defining whether it is for decreasing churn or call center improvement.
Solutions:
Establish quantifiable KPIs, for example, “Decrease manual reporting by 40
Align AI projects with company goals.
Carry out quarterly reviews to monitor progress
Navigating the Path Forward
Transcending AI hype to reality calls for a balanced dual strategy: solving the technology challenges and winning organizational acceptance. Focusing on maintaining data integrity, ethical methodologies, and cross-departmental collaboration will not only help overcome the challenges but also see companies leverage the full capabilities of AI. Pro-active adjustment rather than adoption of the technology will be what differentiates leaders from followers when the technology evolves.
By addressing those barriers head-on, companies can turn AI from a buzz term to a pillar of innovation, promoting growth and durability amid a more competitive environment.So, contact us now, and we will guide you in integrating intelligent automation using AI to make your digital transformation journey smoother.